As Investors become more aware of the potential that artificial intelligence has to offer the field of active asset management, they are also coming to recognize the problems that its use can bring.
A key area of concern is the conflict between the continually expanding big data required by most AI approaches and the necessity to have a consistent, explicable, long-term investment process. With seemingly limitless big data now mushrooming to include questionable AI-generated data, it has become almost impossible to future-proof this data’s ongoing use or usefulness. Plotinus Asset Management in a new white paper presents the case for a fundamental rethink, proposing a Dynamic Deep Neural Network approach using Small Data. The research was published recently in an [ Arvix ] paper.
Less is more: AI Decision-Making using Dynamic Deep Neural Networks for Short-Term Stock Index Prediction introduces a multi-agent deep-learning method which trades in the futures markets based on the S&P 500 Index. The method is not a lab-model as it is currently deployed as part of Plotinus Asset Management’s actively trading Non-Correlated Alpha Controlled Risk Strategy. Rather, the approach is an innovation founded on existing, well-established machine-learning models which sample market prices and associated derivatives in order to decide whether the investment should be long/short or closed (zero exposure) on a day-to-day decision. The research compares this method’s predictive capabilities with conventional AI machine-learning methods and passive benchmark performance.
To address the big data problem, the method deliberately uses Small Data, restricting its inputs to only price and volatility data. The AI’s Dynamic Deep Neural Network architecture enables the method to develop a contextual understanding of market conditions which it can continuously reassess, rather than conventional time-dependent ones.
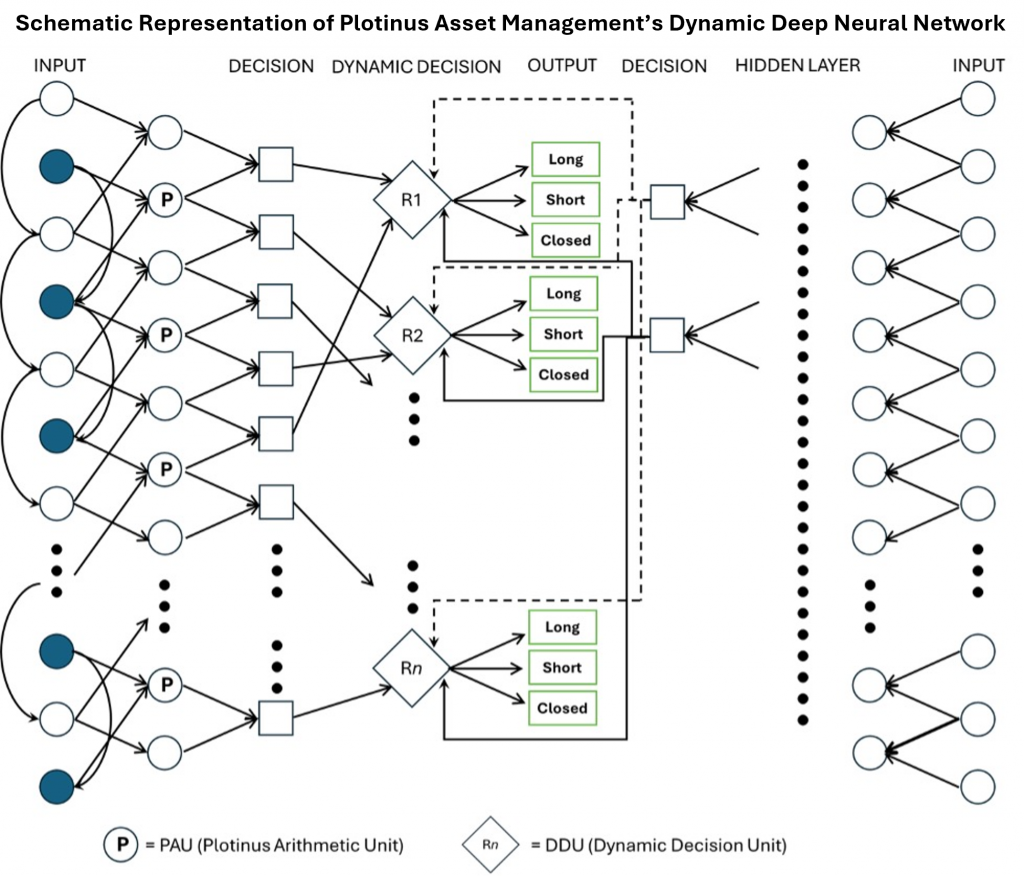
Plotinus’ method is shown to be able to discern when and more importantly when not to trade. Consequently, the method is extremely efficient. In testing it significantly outperformed the passive benchmark by a factor of 4.82, with an exposure to the market of only 41.95% compared to the 100% market exposure of the passive investment. Thus, providing increased profitability with reduced risk. ■
© 2024 Plotinus Asset Management. All rights reserved.
Unauthorized use and/or duplication of any material on this site without written permission is prohibited.
Image Credit: Listiana1979 at Shutterstock.