In examining the impact of Artificial Intelligence in finance, one must look at the potential confusion in defining AI-based-investing and where a lack of explanation inhibits investors from gaining access to and benefits from the technology.
Two Types of Investing
There needs to be some clarity in how to identify when and where Artificial Intelligence is being used as the driver of investment. Two distinct groupings exist: AI decision based and AI assisting-the-process. Many of what could be considered the household names of quantitative or systematic hedge funds are using AI technology in their process. Considering that these firms already have a long tradition in their field, it should be considered that AI is a further tool in the toolkit to help them refine and improve their already established investment process. This perhaps would be best classified as AI assisting-the-process, not specifically AI-driven trading.
Problematically, AI-driven trading is a new field and as a result there are less candidates to consider.
On the other hand, it is not possible to extract the actual benefits that AI is bringing to the investment process at the recognized systematic houses because it is not necessarily helpful to separate it as being distinct from the overall investment approach of the given house.
We therefore have a benchmarking problem. The Eureka Hedge AI index is an attempt to define this challenge, but it clearly runs into the identification problem as expressed above and as a result has adopted quite a broad definition from which to form an index.
This leaves practitioners like ourselves who are using systematic AI decision-based trading with a benchmarking problem. As a result, we think that it is necessary to benchmark by application. The Eureka Hedge AI Index thus serves a function albeit a questionable one for the genre, but this is not particularly helpful for investors who have little awareness or feel for it as a benchmark and who are seeking to understand where the investment benefit of AI lies.
This is where explanation is vital—to provide investors with a context for understanding.
Just Tech Rebranded?
A similar issue is present in the AI investment indices. Take for instance the NASDAQ CTA Artificial Intelligence Index. The measure is composed of companies in the fields of tech/robotics/biomedicine etc. but there is a problem, given the overlap and lack of clear distinction between how these companies benefit from their use of AI.
These types of AI investment indices risk becoming a hodge-podge of many things to many people. AI has enormous promise, but expectation must meet measurable delivery—it is this need to identify defined, restricted expectation that we feel is the real growth area for AI investment decision making. If you identify what the AI edge is, then you can find more suitable, familiar benchmarks by which to gauge performance and gain investor confidence—which is imperative for any new trading approach.
There is no value in hiding behind the black box. Investors are long past being over-awed by technology and are much more affected by results from its application.
Comparing Apples with Oranges
There is a question as to what should determine a legitimate comparison. Is it the technological approach used in trading? Or should it be by trading style?
Basing a comparison on technological approach, finding purely AI executing approaches is difficult, as investors are limited by both available information and available data. One example that does exist is the US equities trading ETF emanating from use of IBM’s super-computer Watson, the EquBot AI Powered Equity ETF (NYSE: AIEQ).
Bad Is More Relevant Than Good
With a limited field of data, it is sensible to look at the overall context of the market over that period of time. 2019 has been an uncommonly good year for S&P500 returns and this has the unfortunate blurring effect of having the rising tide lifting all ships. Thus, the stock picking merits of any trader cannot be fully verified—this is just as true for the AI executing managers. Within the short timeframe it is more helpful to look at the worst performing period in the market. In recent times, this is exemplified by the slump from September to December 2018. Although short-lived this perhaps may shed light on some AI executing trader’s Achilles heel—potential data overfitting—which can be great when the going is good but can be devastating when times are bad.
Elementary? My Dear Watson
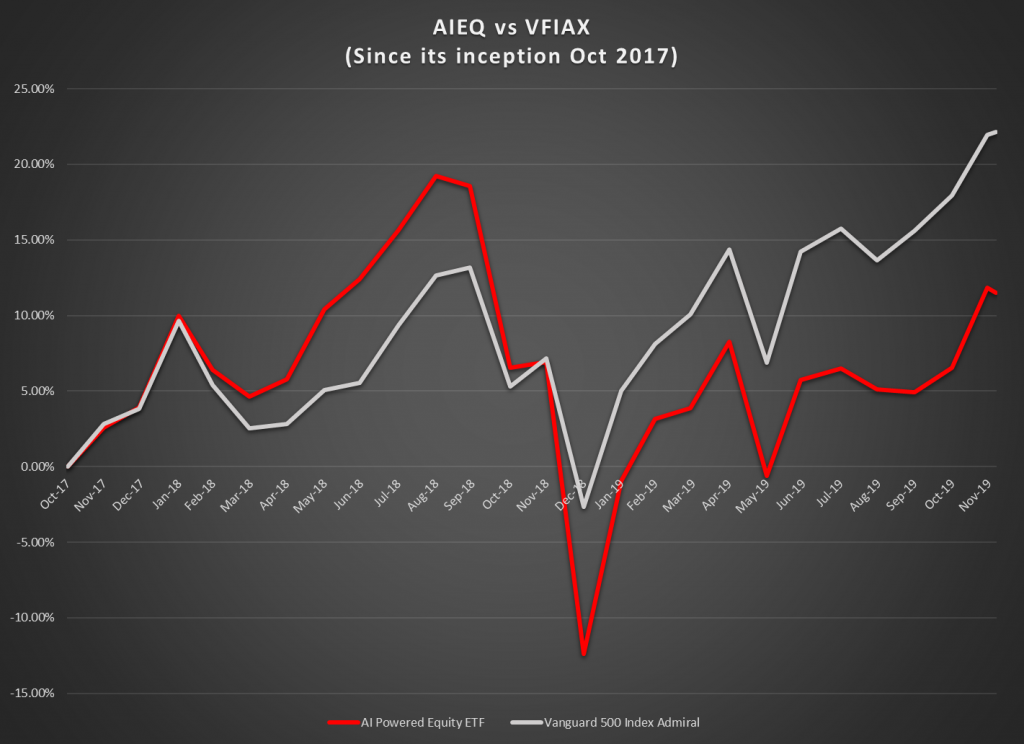
If we look at EquBot’s AIEQ AI Powered Equity ETF you can see that it fell into the September – December 2018 problem and like any active manager the yardstick that they must to measure up against is a passive S&P tracker fund (the chart above shows the Vanguard VFIAX).
VFIAX | ||||
AIEQ | ||||
Note: Data Since Inception Oct 2017. |
You can see that what was a three month -13.97% drawdown for the VFIAX, was a four month -26.53% drawdown for the AIEQ. Furthermore, the additional volatility that the AIEQ brings, 20.35% annualized compared to the VFIAX 14.04% annualized for the period makes the AI stock picker even less attractive.
Slip Sliding Away
The other issue for the stock picking variety of AI executor is when and where does selection start and end? Unless there is a clear, specified investment process with regard to the holdings in these funds, they are susceptible to strategy drift, via a lack of consistency in investment process. Think of their competition, primarily the passive index tracking funds who have a clear rigidly defined investment process, your Vanguard index tracker will not begin the year with 500 constituents and end it with 132 or 694 because it was determined that this would produce a better return.
One of the benefits (if you might call it that) of the limitations of an old-style human stock-picking active manager was that the selection of picks had to remain humanly comprehensible and manageable. Thus, if strategy drift was emerging, it had to be accounted for and explained to investors.
So, the basic question for an AI execution strategy is not; how have you followed the market up in good times? but rather why did you under perform the market by a factor of X when it was doing badly? As can be seen from the chart, matching the market in good times does not compensate for badly under-performing it in its downturn.
Explicable and Comparable
AI has to be conveyed to the investor as a clear helpful assistance to their investing. It must be specific and relatable, not only in performance terms (which is easy to see) but more importantly in investment process terms which is where things can become opaque and inconsistent.
We believe that an AI investment manager must have a strong philosophy in terms of both investment and scientific discipline. This has to take into consideration that the field of AI has a heritage of not settling, as the technology itself continues to advance. There is a temptation to seek increasingly large quantities of data to help, what may be disparagingly described as complex, better curve fitting. Whilst this works in modelling, it does not lend itself so well to the real world of investing where investors really do feel the pain of loss, not a simulated version of it. Their tolerance and fear of uncertainty can be just as important as their tolerance and fear of loss. Investors must be offered task specific AI execution that can illustrate benefits which, given as already mentioned the short track record of these approaches, must show that it is safer than its passive equivalent.
To this end, we have created the Plotinus 2π Enhanced Index which deploys AI execution by trading the S&P 500 Emini Futures. We offer the investor an alternative to their S&P 500 index tracker fund for investors who require the returns but are concerned about their degree of exposure to the index. Our work offers the investor returns similar to those of the tracker fund but does so whilst reducing exposure to the index by 25%.
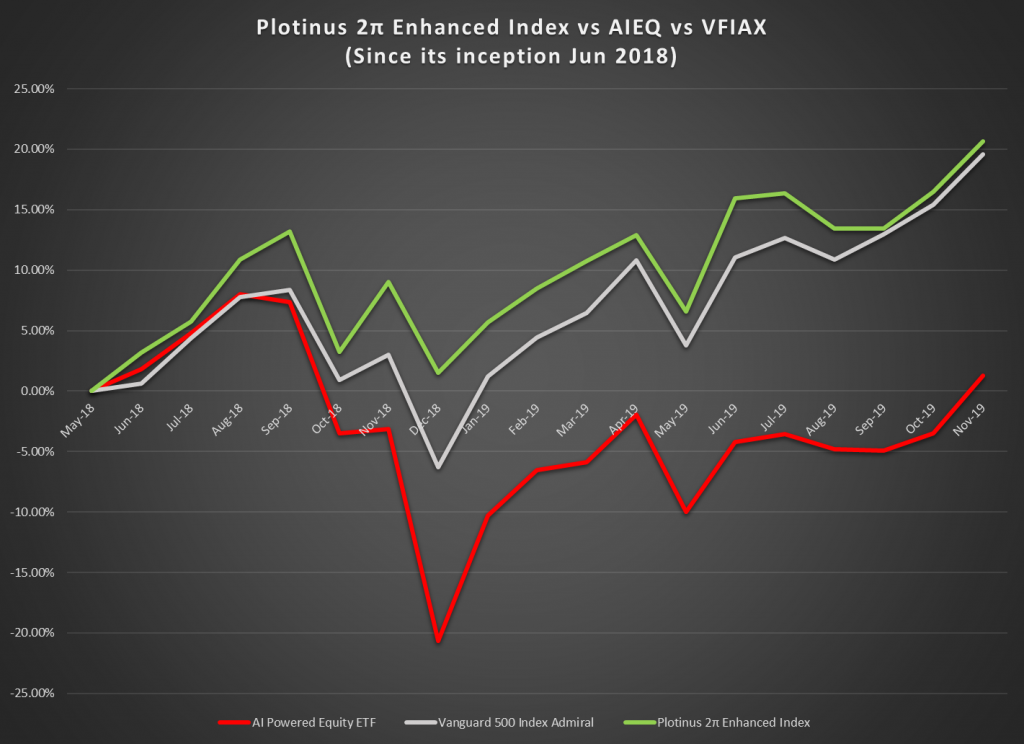
It has the same issue of the short track record like others in the AI execution field – it has an inception date of June 2018 but fortunately it has been through the aforementioned September – December 2018 slump. You can see from the chart the drawdown spans the same three-month period experienced by the S&P 500 but its depth is considerably less at -10.36% than those of the VFIAX and the AIEQ.
We believe what is crucial is that by having a clearly defined investment process our enhanced index does not suffer from the mobility problem of choosing from a varied selection of stocks to be picked, it is able to be genuinely and comfortably compared with the S&P500 Total Return. This means that investors have a process that can be understood but perhaps more significantly has a benchmark that they fully understand. As a result, they can perform a like for like comparison to easily assess whether AI execution as a new technology can offer them measurable investment benefits. ■
© 2019 Plotinus Asset Management LLC. All rights reserved.
Unauthorized use and/or duplication of any material on this site without written permission is prohibited.
Image Credit: Peshkov at Can Stock Photo Inc.